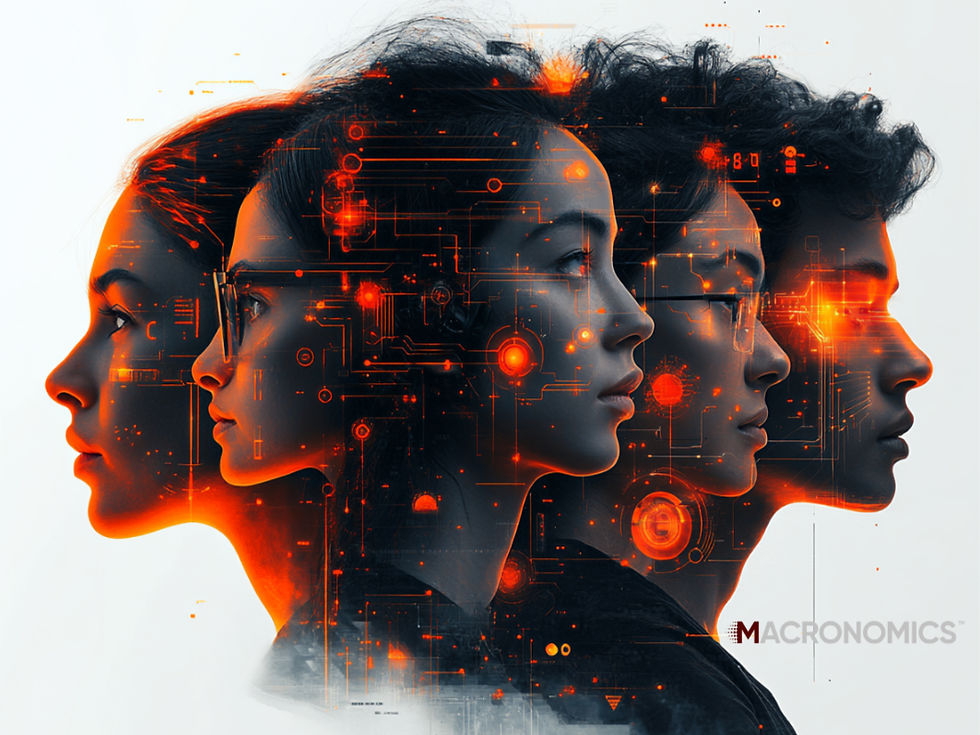
Artificial intelligence (AI) has quickly shifted from being a futuristic concept to a powerful driver of business success. Organizations across industries are leveraging AI to unlock new revenue streams, enhance customer experiences, and streamline operations. But here’s the challenge: while the potential of AI is limitless, its implementation often falls short due to fragmented efforts, siloed teams, and overlooked security risks.
That’s where an AI Center of Excellence (CoE) comes in. A well-structured AI CoE can transform how your organization develops, deploys, and secures AI solutions—ensuring not just innovation but also trust and resilience in an increasingly competitive and security-conscious landscape.
An AI CoE provides the structure, expertise, and governance to ensure AI initiatives deliver measurable value across the organization. While the opportunities are immense, so are the risks, particularly around AI security, data privacy, and ethical usage.
This guide walks senior business leaders through the key steps to create and manage a thriving AI CoE, with a focus on addressing AI security challenges.
________________________________________
What Is an AI Center of Excellence?
An AI CoE is a centralized function that ensures AI efforts align with organizational objectives. It provides leadership, resources, and best practices to support AI initiatives while addressing cross-functional needs like security, compliance, and innovation.
For example, a global logistics firm established an AI CoE to streamline operations with predictive analytics. Without this centralization, the company faced inconsistent implementation across regions, making systems vulnerable to cyber threats. The CoE not only optimized operations but also implemented uniform security protocols to protect sensitive logistics data.
________________________________________
Step 1: Define the Vision and Objectives
A clear vision ensures the CoE focuses on initiatives that align with business priorities while minimizing risks.
• Identify Business Challenges: Are you aiming to improve efficiency, personalize customer experiences, or enhance risk management?
• Set Clear Goals: Define measurable objectives, such as increasing revenue by 15% through AI-powered recommendations or reducing security incidents by 20%.
• Incorporate Security from the Start: Include AI security considerations in your objectives, such as ensuring compliance with data protection laws or preventing adversarial attacks on AI models.
For instance, a healthcare organization launched an AI CoE with the dual goals of improving patient outcomes and ensuring data privacy under HIPAA regulations. This balanced approach to innovation and security was able to solidify stakeholder trust.
________________________________________
Step 2: Build a Multidisciplinary Team
The success of your CoE depends on the expertise and collaboration of its members.
• AI Specialists: Data scientists and engineers who build and optimize AI models.
• Business Analysts: Professionals who ensure AI solutions address real-world business needs.
• IT and Security Experts: Teams responsible for securing AI infrastructure, protecting data, and monitoring systems for vulnerabilities.
• Ethics and Compliance Officers: Advisors to ensure AI use complies with regulations and ethical standards.
For example, a retail company experienced a breach when hackers exploited vulnerabilities in its AI-powered recommendation system. After creating a CoE, the company brought in security specialists to strengthen defenses, significantly reducing risks.
________________________________________
Step 3: Establish Robust AI Infrastructure
The right infrastructure supports secure, scalable AI operations.
• Cloud Platforms and Tools: Use secure and scalable cloud environments like AWS, Azure, Oracle Cloud Infrastructure, Google Cloud, or other leaders. Opt for providers offering AI- specific security features, such as encryption for model training and deployment.
• Data Protection Systems: Implement tools that encrypt data both in transit and at rest. Regularly update and patch software to address vulnerabilities.
• Secure Model Development Pipelines: Use frameworks like TensorFlow and PyTorch, combined with security tools to protect models from adversarial attacks.
AI systems are increasingly targeted by bad actors through methods like data poisoning (introducing malicious data into training sets) or model inversion (reverse-engineering sensitive data). Proactively mitigating these threats is essential.
________________________________________
Step 4: Governance and Ethical Standards
Governance frameworks ensure that AI initiatives are transparent, ethical, and secure.
• Develop AI Security Policies: Include protocols for detecting, responding to, and recovering from AI-specific attacks, such as adversarial inputs or model theft.
• Establish Ethical Guidelines: Define principles for fairness, transparency, and accountability in AI usage.
• Monitor for Bias and Misuse: Regularly audit AI models to detect biases and vulnerabilities that could compromise security or trust.
• Ensure Regulatory Compliance: Stay updated on global AI regulations, such as GDPR, HIPAA, or the AI Act, and ensure your CoE meets these standards.
A financial institution, for instance, established governance policies to prevent bias in credit-scoring models while implementing systems to monitor fraud and prevent attacks on AI decision-making systems.
________________________________________
Step 5: Prioritize Security in Early Projects
Launching initial projects with strong security practices sets the tone for the CoE’s future.
• Start with Contained Use Cases: Pilot AI in areas with manageable data sensitivity, such as internal process optimization, before expanding to customer-facing applications.
• Incorporate Threat Modeling: Assess potential risks during the design phase to prevent vulnerabilities later.
• Test Security Protocols: Conduct penetration testing to identify weaknesses in AI systems.
For instance, a manufacturing firm piloted an AI system for predictive maintenance, ensuring it met security benchmarks before expanding its use across multiple facilities. This phased approach minimized risks while demonstrating value.
________________________________________
Step 6: Build a Collaborative and Security-Conscious Culture
AI success depends on fostering collaboration across departments and embedding security into the organization's DNA.
• Educate Employees: Provide training on AI fundamentals and security best practices to all stakeholders, including non-technical staff.
• Encourage Knowledge Sharing: Create channels for CoE members to share insights, such as lessons learned from addressing cyber threats.
• Promote Accountability: Assign clear ownership for AI security, ensuring someone is responsible for monitoring and mitigating risks.
A retail company cultivated a culture of AI security by sharing examples of cyberattacks on AI systems during company-wide meetings, helping employees recognize the importance of secure practices.
________________________________________
Step 7: Measure, Adapt, and Evolve
Continuous improvement is key to managing an AI CoE effectively.
• Monitor Key Metrics: Track business outcomes, AI performance, and security incidents.
• Regularly Audit Security Practices: Review and update security protocols as threats evolve.
• Adapt to New Technologies: Stay informed about advancements in AI and cybersecurity, incorporating them into your CoE.
For example, a tech company faced an adversarial attack on its AI fraud detection system. By adapting its security protocols and retraining its model, the CoE not only mitigated the issue but also enhanced the system’s resilience against future threats.
________________________________________
Key Benefits of an AI CoE with Strong Security Practices
Building an AI CoE with security at its core delivers tangible benefits:
• Scalability: Secure systems enable consistent, enterprise-wide AI adoption.
• Trust: Customers and stakeholders feel confident in systems designed to protect data and ensure compliance.
• Resilience: Proactive security measures safeguard AI assets from emerging threats.
• Efficiency: Centralized governance reduces inefficiencies and duplication of effort.
________________________________________
Conclusion: Building a Secure and Scalable AI Future
An AI Center of Excellence is your organization’s hub for innovation and operational excellence. However, in today’s threat landscape, security must be a foundational element of your CoE. By centralizing expertise, fostering a culture of collaboration, and prioritizing security at every step, you can build AI systems that are not only innovative but also resilient and trustworthy.
Take action today: Define your vision, build your team, and integrate security as a non-negotiable pillar of your AI CoE. This approach ensures your organization stays competitive, compliant, and prepared for the future. Contact us anytime for a conversation about how we can help!
Comments